Projects
The Kuenneth group works on all four pillars of materials informatics. The picture below shows these four pillars
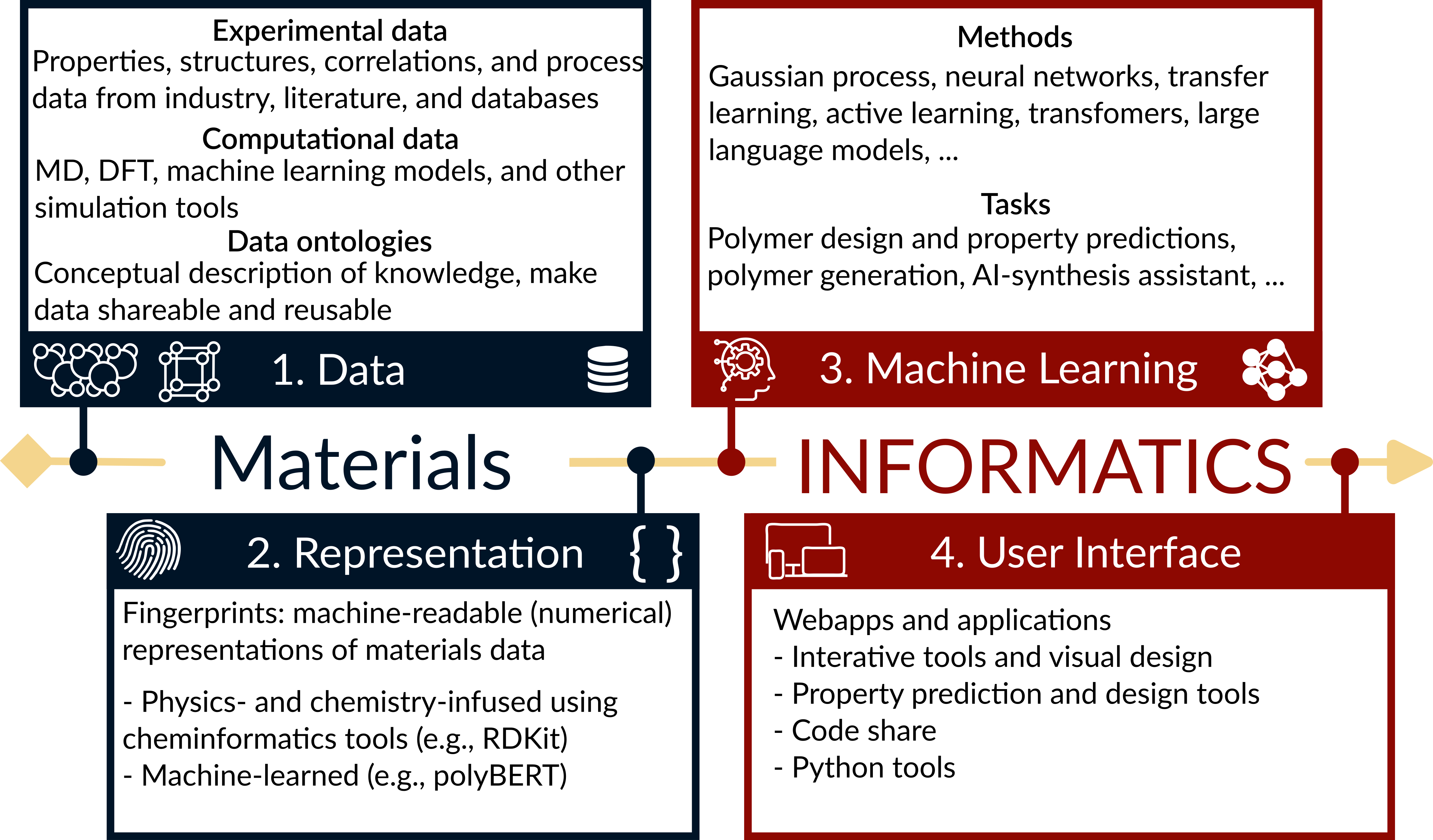
Sample projects
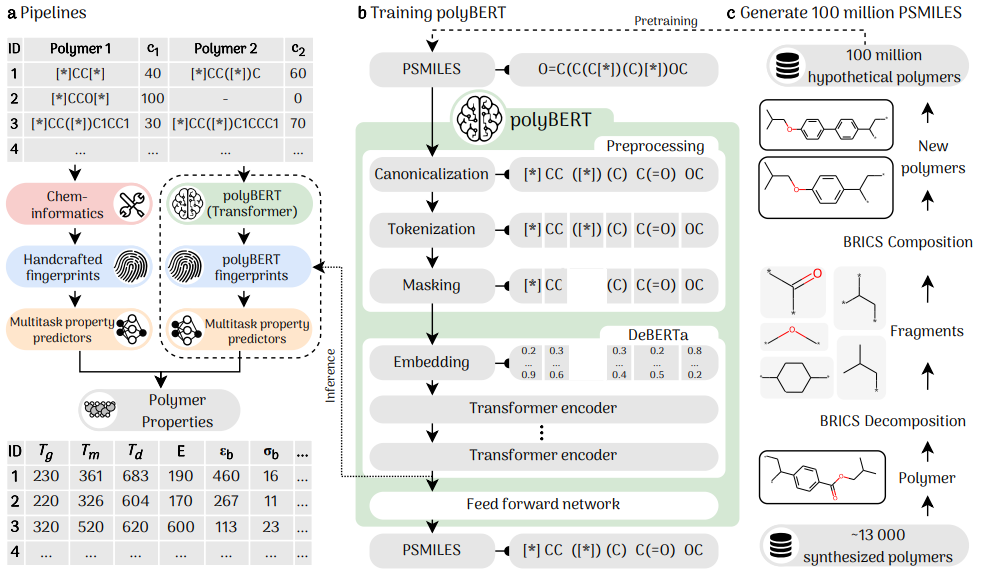
polyBERT: A chemical language model to enable fully machine-driven ultrafast polymer informatics
Polymers are a vital part of everyday life. Their chemical universe is so large that it presents unprecedented opportunities as well as significant challenges to identify suitable application-specific candidates. We present a complete end-to-end machine-driven polymer informatics pipeline that can search this space for suitable candidates at unprecedented speed and accuracy. This pipeline includes a polymer chemical fingerprinting capability called polyBERT (inspired by Natural Language Processing concepts), and a multitask learning approach that maps the polyBERT fingerprints to a host of properties. polyBERT is a chemical linguist that treats the chemical structure of polymers as a chemical language. The present approach outstrips the best presently available concepts for polymer property prediction based on handcrafted fingerprint schemes in speed by two orders of magnitude while preserving accuracy, thus making it a strong candidate for deployment in scalable architectures including cloud infrastructures.
C. Kuenneth, R. Ramprasad, polyBERT: A chemical language model to enable fully machine-driven ultrafast polymer informatics
Link to arXiv
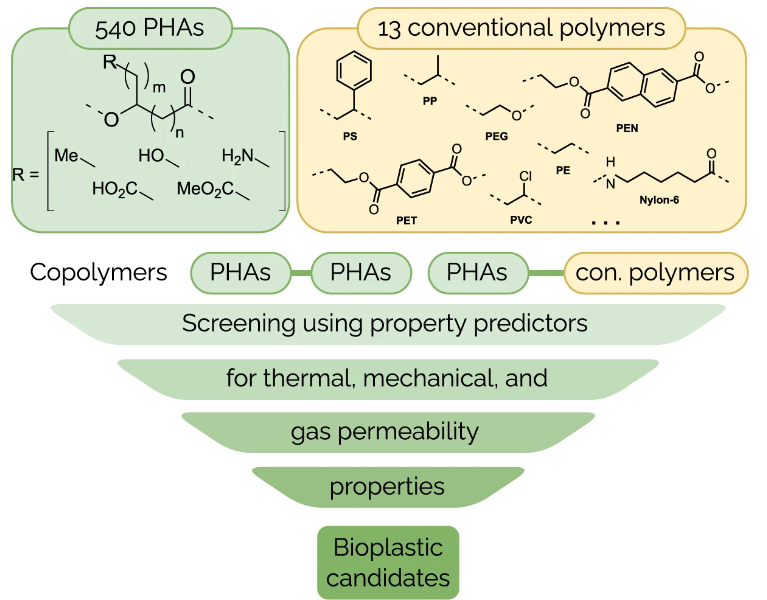
Bioplastic design using multitask deep neural networks
Non-degradable plastic waste stays for decades on land and in water, jeopardizing our environment; yet our modern lifestyle and current technologies are impossible to sustain without plastics. Bio-synthesized and biodegradable alternatives such as the polymer family of polyhydroxyalkanoates (PHAs) have the potential to replace large portions of the world's plastic supply with cradle-to-cradle materials, but their chemical complexity and diversity limit traditional resource-intensive experimentation. In this work, we develop multitask deep neural network property predictors using available experimental data for a diverse set of nearly 23000 homo- and copolymer chemistries. Using the predictors, we identify 14 PHA-based bioplastics from a search space of almost 1.4 million candidates which could serve as potential replacements for seven petroleum-based commodity plastics that account for 75% of the world's yearly plastic production. We discuss possible synthesis routes for these identified promising materials.
C. Kuenneth, J. Lalonde, B.L. Marrone, C.N. Iverson, R. Ramprasad, G. Pilania. Bioplastic design using multitask deep neural networks. Commun Mater 3, 96 (2022). DOI: 10.1038/s43246-022-00319-2
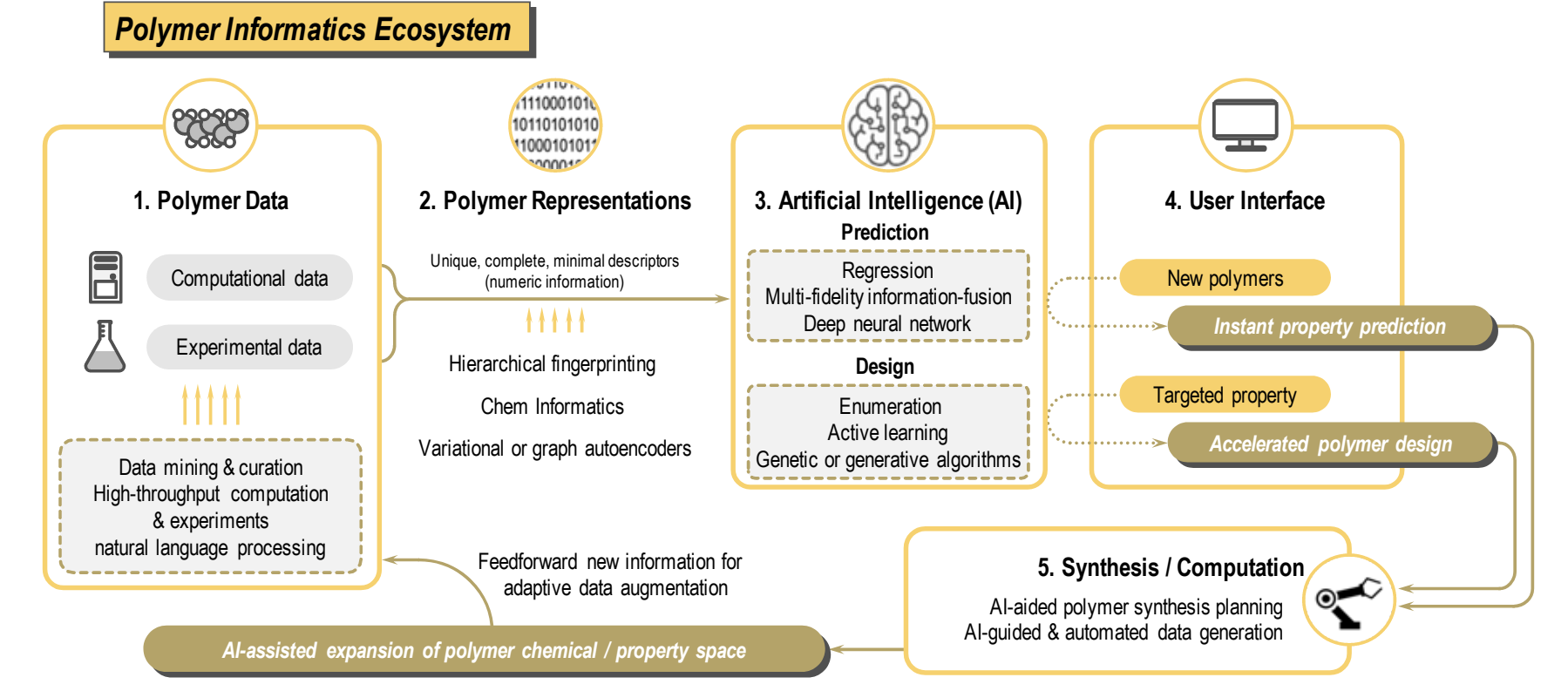
Polymer informatics: Current status and critical next steps
Artificial intelligence (AI) based approaches are beginning to impact several domains of human life, science and technology. Polymer informatics is one such domain where AI and machine learning (ML) tools are being used in the efficient development, design and discovery of polymers. Surrogate models are trained on available polymer data for instant property prediction, allowing screening of promising polymer candidates with specific target property requirements. Questions regarding synthesizability, and potential (retro)synthesis steps to create a target polymer, are being explored using statistical means. Data-driven strategies to tackle unique challenges resulting from the extraordinary chemical and physical diversity of polymers at small and large scales are being explored. Other major hurdles for polymer informatics are the lack of widespreadavailability of curated and organized data, and approaches to create machine-readable representations that capture not just the structure of complex polymeric situations but also synthesis and processing conditions. Methods to solve inverse problems, wherein polymer recommendations are made using advanced AI algorithms that meet application targets, are being investigated. As various parts of the burgeoning polymer informatics ecosystem mature and become integrated, efficiency improvements, accelerated discoveries and increased productivity can result. Here, we review emergent components of this polymer informatics ecosystem and discuss imminent challenges and opportunities.
L. Chen, G. Pilania, R. Batra, T.D. Huan, C. Kim, C. Kuenneth, R. Ramprasad, “Polymer informatics: Current status and critical next steps”, Materials Science and Engineering: R: Reports 2021, 144, 100595. DOI: 10.1016/j.mser.2020.100595
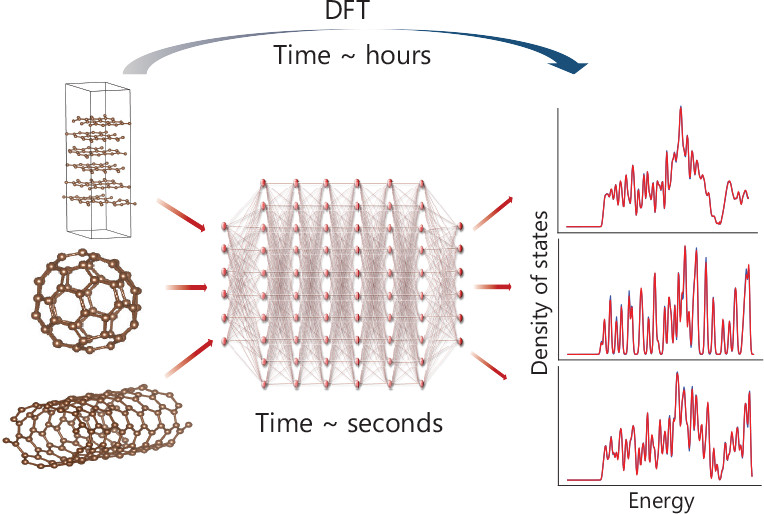
An efficient deep learning scheme to predict the electronic structure of materials and molecules: The example of graphene-derived allotropes
Computations based on density functional theory (DFT) are transforming various aspects of materials research and discovery. However, the effort required to solve the central equation of DFT, namely the Kohn–Sham equation, which remains a major obstacle for studying large systems with hundreds of atoms in a practical amount of time with routine computational resources. Here, we propose a deep learning architecture that systematically learns the input–output behavior of the Kohn–Sham equation and predicts the electronic density of states, a primary output of DFT calculations, with unprecedented speed and chemical accuracy. The algorithm also adapts and progressively improves in predictive power and versatility as it is exposed to new diverse atomic configurations. We demonstrate this capability for a diverse set of carbon allotropes spanning a large configurational and phase space. The electronic density of states, along with the electronic charge density, may be used downstream to predict a variety of materials properties, bypassing the Kohn–Sham equation, leading to an ultrafast and high-fidelity DFT emulator.
Beatriz G. del Rio, Christopher Kuenneth, Huan Doan Tran, and Rampi Ramprasad "An Efficient Deep Learning Scheme To Predict the Electronic Structure of Materials and Molecules: The Example of Graphene-Derived Allotropes", The Journal of Physical Chemistry A 2020 124 (45), 9496-9502, DOI: 10.1021/acs.jpca.0c07458
Link to article